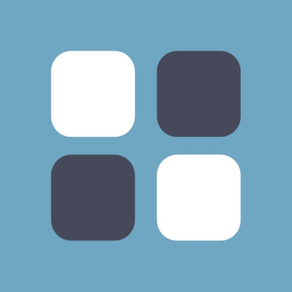
Confusion Matrix Calculator
Free
1.0for iPhone, iPad
Age Rating
Confusion Matrix Calculator Capturas de tela
About Confusion Matrix Calculator
This Confusion Matrix Calculator determines several statistical measures linked to the performance of classification models such as: Sensitivity, Specificity, Positive Predictive Value (Precision), Negative Predictive Value, False Positive Rate, False Discovery Rate, False Negative Rate, Accuracy & Matthews Correlation Coefficient.
Statistical measures based on the confusion matrix
The confusion matrix is the popular representation of the performance of classification models and includes the correctly and incorrectly classified values compared to the actual outcomes in the test data. The four variables are:
True positive (TP) – which is the outcome where the model correctly predicts positive class (condition is correctly detected when present);
True negative (TN) – which is the outcome where the model correctly predicts negative class (condition is not detected when absent);
False positive (FP) – which is the outcome where the model incorrectly predicts positive class (condition is detected despite being absent);
False negative (FN) – which is the outcome where the model incorrectly predicts negative class (condition is not detected despite being present).
One of the most commonly determined statistical measures is Sensitivity (also known as recall, hit rate or true positive rate TPR). Sensitivity measures the proportion of actual positives that are correctly identified as positives.
Sensitivity = TP / (TP + FN)
Specificity, also known as selectivity or true negative rate (TNR), measures the proportion of actual negatives that are correctly identified as negatives.
Specificity = TN / (FP + TN)
The Positive Predictive Value (PPV), also known as Precision and the Negative Predictive Value (NPV) are the proportion of positive and negative results that are true positive, respectively true negative. They are also called positive respectively negative predictive agreements and are measures of the performance of a diagnostic test.
Positive Predictive Value (Precision) = TP / (TP + FP)
Negative Predictive Value = TN / (TN + FN)
The False Positive Rate (FPR) or fall-out is the ratio between the number of negative events incorrectly categorized as positive (false positives) and the total number of actual negative events (regardless of classification).
False Positive Rate = FP / (FP + TN)
The False Discovery Rate (FDR) is a statistical approach used in multiple hypothesis testing to correct for multiple comparisons.
False Discovery Rate = FP / (FP + TP)
The False Negative Rate (FNR) measures the proportion of the individuals where a condition is present for which the test result is negative.
False Negative Rate = FN / (FN + TP)
Accuracy (ACC) is a measure of statistical bias
Accuracy = (TP + TN) / (TP + TN + FP + FN)
The F1 Score is a measure of a test’s accuracy, defined as the harmonic mean of precision and recall.
F1 Score = 2TP / (2TP + FP + FN)
Matthews Correlation Coefficient (MCC) describes how changing the value of one variable will affect the value of another and returns a value between -1 and 1:
+1 describes a perfect prediction;
0 unable to return any valid information (no better than random prediction);
-1 describes complete inconsistency between prediction and observation.
Matthews Correlation Coefficient = (TP x TN – FP x FN) / (sqrt((TP+FP) x (TP+FN) x (TN+FP) x (TN+FN)))
Disclaimer: Always seek a doctor’s advice in addition to using this app and before making any medical decisions. This app should NOT be considered as a substitute for professional medical service, NOR as a substitute for clinical judgement.
Statistical measures based on the confusion matrix
The confusion matrix is the popular representation of the performance of classification models and includes the correctly and incorrectly classified values compared to the actual outcomes in the test data. The four variables are:
True positive (TP) – which is the outcome where the model correctly predicts positive class (condition is correctly detected when present);
True negative (TN) – which is the outcome where the model correctly predicts negative class (condition is not detected when absent);
False positive (FP) – which is the outcome where the model incorrectly predicts positive class (condition is detected despite being absent);
False negative (FN) – which is the outcome where the model incorrectly predicts negative class (condition is not detected despite being present).
One of the most commonly determined statistical measures is Sensitivity (also known as recall, hit rate or true positive rate TPR). Sensitivity measures the proportion of actual positives that are correctly identified as positives.
Sensitivity = TP / (TP + FN)
Specificity, also known as selectivity or true negative rate (TNR), measures the proportion of actual negatives that are correctly identified as negatives.
Specificity = TN / (FP + TN)
The Positive Predictive Value (PPV), also known as Precision and the Negative Predictive Value (NPV) are the proportion of positive and negative results that are true positive, respectively true negative. They are also called positive respectively negative predictive agreements and are measures of the performance of a diagnostic test.
Positive Predictive Value (Precision) = TP / (TP + FP)
Negative Predictive Value = TN / (TN + FN)
The False Positive Rate (FPR) or fall-out is the ratio between the number of negative events incorrectly categorized as positive (false positives) and the total number of actual negative events (regardless of classification).
False Positive Rate = FP / (FP + TN)
The False Discovery Rate (FDR) is a statistical approach used in multiple hypothesis testing to correct for multiple comparisons.
False Discovery Rate = FP / (FP + TP)
The False Negative Rate (FNR) measures the proportion of the individuals where a condition is present for which the test result is negative.
False Negative Rate = FN / (FN + TP)
Accuracy (ACC) is a measure of statistical bias
Accuracy = (TP + TN) / (TP + TN + FP + FN)
The F1 Score is a measure of a test’s accuracy, defined as the harmonic mean of precision and recall.
F1 Score = 2TP / (2TP + FP + FN)
Matthews Correlation Coefficient (MCC) describes how changing the value of one variable will affect the value of another and returns a value between -1 and 1:
+1 describes a perfect prediction;
0 unable to return any valid information (no better than random prediction);
-1 describes complete inconsistency between prediction and observation.
Matthews Correlation Coefficient = (TP x TN – FP x FN) / (sqrt((TP+FP) x (TP+FN) x (TN+FP) x (TN+FN)))
Disclaimer: Always seek a doctor’s advice in addition to using this app and before making any medical decisions. This app should NOT be considered as a substitute for professional medical service, NOR as a substitute for clinical judgement.
Show More
Novidades da Última Versão 1.0
Last updated on Dec 2, 2021
Version History
1.0
Dec 2, 2021
Confusion Matrix Calculator FAQ
Clique aqui para saber como baixar Confusion Matrix Calculator em um país ou região restrita.
Confira a lista a seguir para ver os requisitos mínimos de Confusion Matrix Calculator.
iPhone
iPad
Confusion Matrix Calculator suporta English